AI improves diagnosis, reduces false positives from mammo images
Radiologists getting an assist from artificial intelligence can detect more breast cancer—with a reduced rate of false positive incidents—from mammography images.
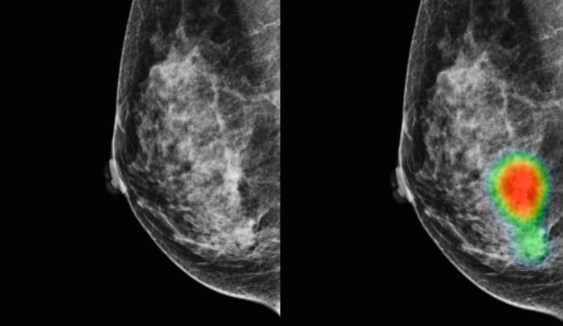
Mammograms of a 49-year-old woman with invasive lobular carcinoma on the right-side breast. A small mass with micro-calcifications on the right-side breast was detected correctly by AI with an abnormality score of 96%. This case was recalled by 7 out of 14 radiologists (4 breast radiologists and 3 general radiologists) initially (without AI) and all […]
More for you
See AllLoading data for hdm_tax_topic #better-outcomes...